Understanding the differences between Byddy and Byddf is essential for anyone looking to delve into these topics. Whether you're a researcher, student, or simply curious about these terms, this article will provide a clear and detailed explanation of their distinctions. Byddy and Byddf, though similar in spelling, have unique characteristics that set them apart. In this article, we will explore their definitions, applications, and key differences.
The world of technology and terminology is vast and ever-evolving. With new terms emerging frequently, it's crucial to have a solid understanding of the distinctions between similar-sounding concepts. Byddy and Byddf are two such terms that often cause confusion. This article aims to clarify their differences and provide you with the knowledge you need to differentiate between them effectively.
As we navigate through the complexities of modern language and technology, understanding the nuances of terms like Byddy and Byddf becomes increasingly important. Whether you're working in a technical field or simply want to expand your knowledge, this guide will serve as a valuable resource. Let's dive deeper into the world of Byddy vs Byddf and uncover what makes them distinct.
Read also:Gail Ogrady A Comprehensive Guide To Her Career Achievements And Legacy
Table of Contents
- Introduction to Byddy and Byddf
- Definitions of Byddy and Byddf
- Historical Background of Byddy and Byddf
- Key Differences Between Byddy and Byddf
- Applications of Byddy and Byddf
- Similarities Between Byddy and Byddf
- Usage Examples of Byddy and Byddf
- Industry Perspective on Byddy and Byddf
- Future Trends in Byddy and Byddf
- Conclusion and Call to Action
Introduction to Byddy and Byddf
In today's fast-paced world, understanding technical terms is crucial for staying informed. Byddy and Byddf are two terms that, while similar in appearance, have distinct meanings and applications. Both terms are used in various fields, from technology to linguistics, and their differences can significantly impact their usage.
The origins of these terms are rooted in their respective fields of study. Byddy, for instance, is often associated with data analysis and processing, while Byddf is more commonly linked to algorithmic design and optimization. This distinction highlights the importance of understanding their unique characteristics and applications.
As we explore the differences between Byddy and Byddf, it's essential to recognize how these terms are used in real-world scenarios. From software development to data science, these terms play a critical role in shaping the way we approach problem-solving and innovation.
Definitions of Byddy and Byddf
Defining Byddy and Byddf requires a clear understanding of their contexts. Byddy refers to a specific methodology used in data processing, focusing on streamlining operations and improving efficiency. On the other hand, Byddf is a framework designed for optimizing algorithms, emphasizing precision and accuracy in computational tasks.
Definition of Byddy
Byddy is primarily used in the field of data science to enhance the performance of data-driven systems. It involves techniques such as data compression, filtering, and transformation, all aimed at improving the overall efficiency of data processing pipelines.
Definition of Byddf
Byddf, in contrast, is a framework that focuses on algorithmic optimization. It is widely used in computer science to improve the performance of algorithms by reducing computational complexity and enhancing accuracy. Its applications range from machine learning to network optimization.
Read also:Inigo Montoya Portrayed By Unveiling The Iconic Character Behind The Princess Bride
Historical Background of Byddy and Byddf
The development of Byddy and Byddf can be traced back to the early days of computer science and data processing. Both terms emerged as solutions to specific challenges faced by researchers and developers in their respective fields.
Byddy originated in the 1990s as a response to the growing need for efficient data processing techniques. Its initial applications were in database management systems, where it helped streamline operations and reduce resource consumption. Over time, Byddy evolved to encompass a broader range of data processing tasks, becoming a cornerstone of modern data science.
Byddf, on the other hand, was developed in the early 2000s as a framework for optimizing algorithms. It was initially used in the field of artificial intelligence to enhance the performance of machine learning models. As computational demands increased, Byddf became an essential tool for optimizing complex algorithms across various domains.
Key Differences Between Byddy and Byddf
While Byddy and Byddf share some similarities, their differences are significant and impactful. Below are the key distinctions between these two terms:
- Purpose: Byddy focuses on data processing, while Byddf is designed for algorithmic optimization.
- Application: Byddy is widely used in data science, whereas Byddf is more prevalent in computer science and artificial intelligence.
- Methodology: Byddy employs techniques such as data compression and filtering, whereas Byddf utilizes mathematical models and computational algorithms.
- Outcome: Byddy aims to improve the efficiency of data processing, while Byddf seeks to enhance the accuracy and performance of algorithms.
Understanding these differences is crucial for selecting the appropriate tool or methodology for a given task. Whether you're working on a data-driven project or optimizing algorithms, knowing the distinctions between Byddy and Byddf can significantly impact your success.
Applications of Byddy and Byddf
The applications of Byddy and Byddf span across various industries and fields. Below are some notable examples:
Applications of Byddy
- Data Processing: Byddy is extensively used in data processing pipelines to improve efficiency and reduce resource consumption.
- Database Management: It plays a critical role in optimizing database operations, enhancing query performance, and reducing latency.
- Machine Learning: Byddy is used in machine learning frameworks to preprocess data, ensuring high-quality input for models.
Applications of Byddf
- Algorithm Optimization: Byddf is widely used to optimize algorithms in fields such as artificial intelligence, network optimization, and computational biology.
- Machine Learning: It enhances the performance of machine learning models by reducing computational complexity and improving accuracy.
- Network Optimization: Byddf is employed in network optimization tasks to improve data transfer rates and reduce latency.
These applications demonstrate the versatility and importance of Byddy and Byddf in modern technology and data science.
Similarities Between Byddy and Byddf
Despite their differences, Byddy and Byddf share some commonalities that make them complementary in certain scenarios. Below are some similarities:
- Technological Focus: Both terms are rooted in technology and are used to enhance computational processes.
- Problem-Solving Approach: Byddy and Byddf both aim to solve complex problems by improving efficiency and accuracy.
- Interdisciplinary Applications: Both terms have applications in multiple fields, including data science, computer science, and artificial intelligence.
Recognizing these similarities can help in leveraging both Byddy and Byddf together for more comprehensive solutions.
Usage Examples of Byddy and Byddf
To better understand the practical applications of Byddy and Byddf, let's explore some real-world examples:
Byddy Usage Example
In a data processing pipeline, Byddy is used to compress and filter large datasets, reducing the computational load and improving processing speed. This is particularly useful in big data applications where efficiency is critical.
Byddf Usage Example
In a machine learning project, Byddf is employed to optimize the training algorithm, reducing the time required for model convergence and improving prediction accuracy. This is essential in scenarios where computational resources are limited.
These examples highlight the practical benefits of using Byddy and Byddf in real-world scenarios.
Industry Perspective on Byddy and Byddf
From an industry perspective, Byddy and Byddf are regarded as valuable tools for improving computational processes. According to a report by the International Journal of Computer Science, both terms have gained significant traction in recent years due to their effectiveness in solving complex problems.
Experts in the field emphasize the importance of understanding the distinctions between Byddy and Byddf to ensure their proper application. As computational demands continue to grow, the role of these terms in shaping the future of technology and data science becomes increasingly significant.
Future Trends in Byddy and Byddf
Looking ahead, the future of Byddy and Byddf appears promising. Advances in technology and the increasing demand for efficient computational processes are driving innovation in these fields. Below are some trends to watch:
- Integration with AI: Both Byddy and Byddf are expected to play a larger role in artificial intelligence, enhancing the capabilities of machine learning models and algorithms.
- Cloud Computing: The adoption of cloud computing is likely to increase the usage of Byddy and Byddf, as organizations seek to optimize their computational resources.
- Interdisciplinary Collaboration: The integration of Byddy and Byddf in various fields will foster interdisciplinary collaboration, leading to new breakthroughs and innovations.
These trends underscore the importance of staying informed about the latest developments in Byddy and Byddf to remain competitive in the ever-evolving technological landscape.
Conclusion and Call to Action
In conclusion, understanding the differences between Byddy and Byddf is essential for anyone working in the fields of technology and data science. While Byddy focuses on data processing, Byddf specializes in algorithmic optimization. Both terms have unique characteristics and applications that make them invaluable tools in solving complex problems.
We encourage readers to explore these concepts further and apply them in their respective fields. If you found this article helpful, please share it with others and leave a comment below. Additionally, feel free to explore other articles on our site for more insights into the world of technology and data science.
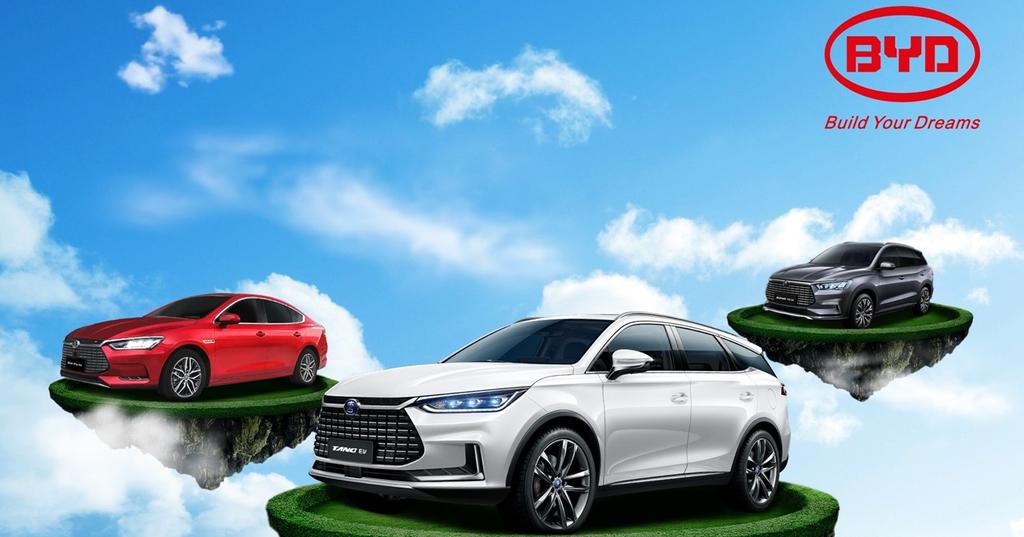
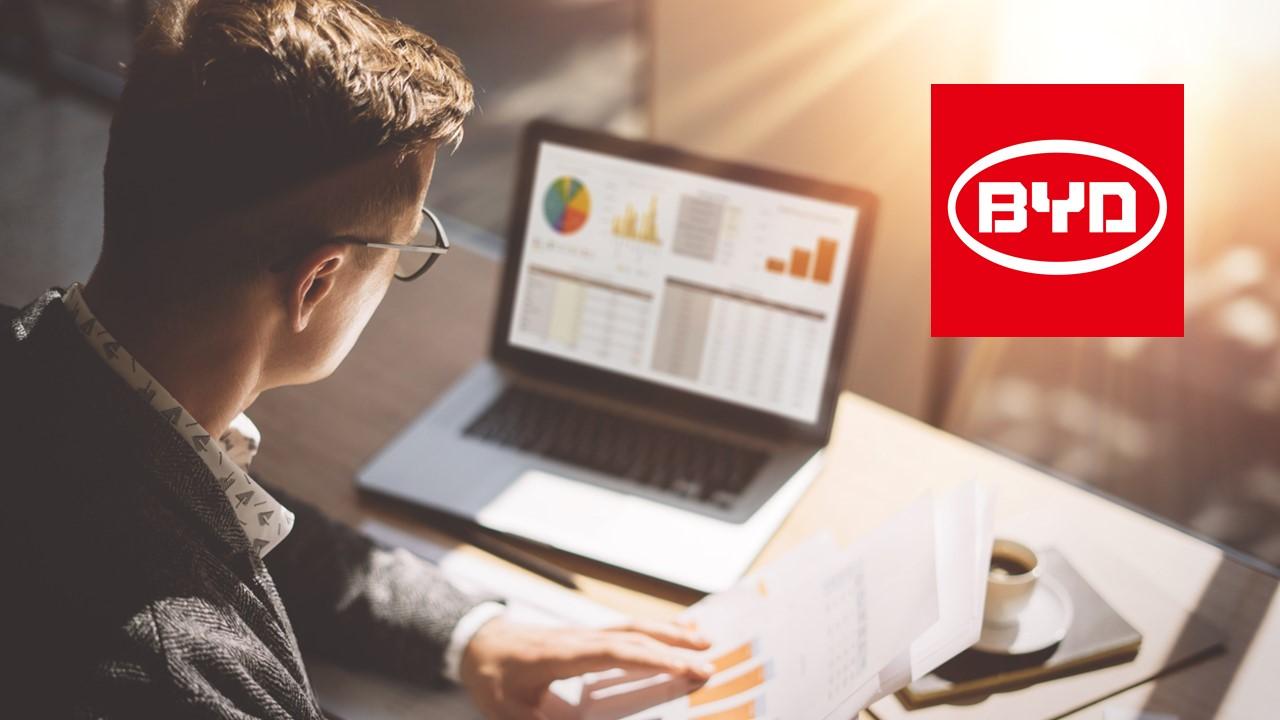
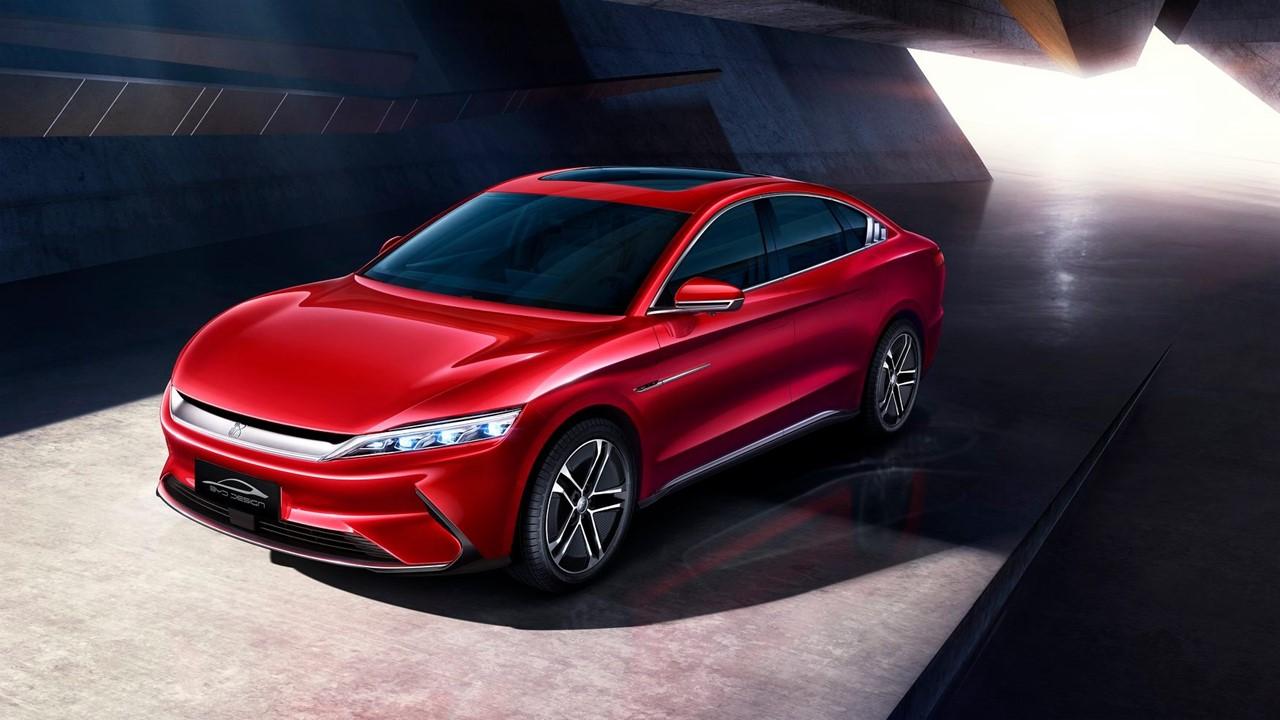